As part of the launch of the new Loihi 2 chip, built on a pre-production version of Intel’s 4 process node, the Intel Labs team behind its Neuromorphic efforts reached out for a chance to speak to Mike Davies, the Director of the project. Now it is perhaps no shock that Intel’s neuromorphic efforts have been on my radar for a number of years – as a new paradigm of computing compared to the traditional von Neumann architecture, and one that is meant to mimic brains and take advantages of such designs, if it works well then it has the potential to shake up specific areas of the industry, as well as Intel’s bottom line. Also, given that we’ve never really covered Neuromorphic computing in any serious detail here on AnandTech, it would be a great opportunity to get details on this area of research, as well as the newest hardware, direct from the source.
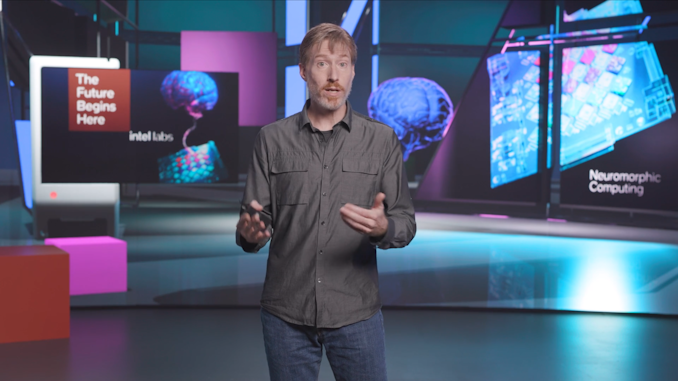
Mike Davies currently sits as Director of Intel’s Neuromorphic Computing Lab, a position held since 2017, as well as having been a principle engineer on the same project. Mike joined Intel in 2011 as part of the acquisition of Fulcrum Microsystems, where he had been in IC development for 11 years. Fulcrum’s focus was on asynchronous network switch design, and after Intel made the acquisition, that technology eventually made its way into Intel’s networking division, and so the asynchronous compute team pivoted to Neuromorphic designs. Mike has been the face of Intel’s Neuromorphic efforts, demonstrating the technology and the extent of the research and collaborations with industry partners and academic institutions at industry events.
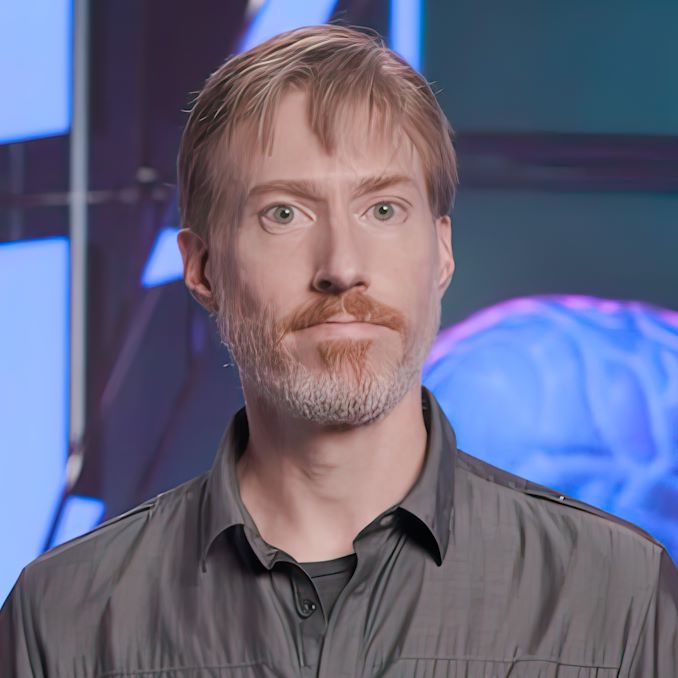
Mike Davies
Director, Intel Labs |
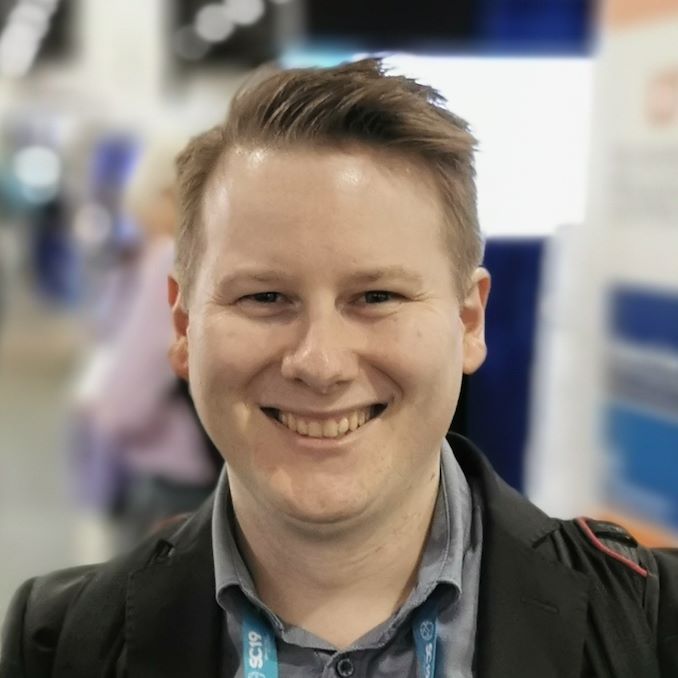
Dr. Ian Cutress
AnandTech |
Ian Cutress: Can you describe what Neuromorphic Computing is, and what it means for Intel?
Mike Davies: Neuromorphic Computing is a rethinking of computer architecture, inspired by the principles of brains. It is really informed at a very low level of our understanding of neuroscience, and it leads us to an architecture that looks dramatically different from even the latest AI accelerators or deep learning accelerators.
It is a fully integrated memory and compute model, so you have computing elements sitting very close to the storage state elements that correspond to the neural state and the synaptic state that represents the network that you're computing. It’s not [a traditional] kind of streaming data model always executing through off chip memory - the data is staying locally, not moving around, until there's something important to be computed. [At that point] the local circuit activates and sends an event based message, or a spike, to all the other neurons that are paying attention to it.
Probably the most fundamental difference to conventional architectures is that the computing process is kind of an emergent phenomenon. All of these neurons can be configured, and they operate as a dynamic system, which means that they evolve over time – and you may not know the precise sequence of instructions or states that they step through to arrive at the solution as you do in a conventional model. It's a dynamic process. You proceed through some collective interaction, and then settle into some new equilibrium state, which is the solution that you're looking for.
So in some ways it has parallels to quantum computing which is also computing with physical interactions between its elements. But here we are dealing with digital circuits, still designed in a pretty traditional way with traditional process technology, but the way we've constructed those circuits, and the architecture overall, is very different from conventional processors.
As far as Intel's outlook, we're hoping that through this research programme, we can uncover a new technology that augments our portfolio of current processors, tools, techniques, and technologies that we have available to us to go and address a wide range of different workloads. This is for applications where we want to deploy really adaptive and intelligent behavior. You can think of anything that moves, or anything that's out in the real world, faces power constraints and latency constraints, and whatever compute is there has to deal with the unpredictability and the variability of the real world. [The compute] has to able to make those adjustments, and respond to data in real time, in a very fast but low power mode of operation.
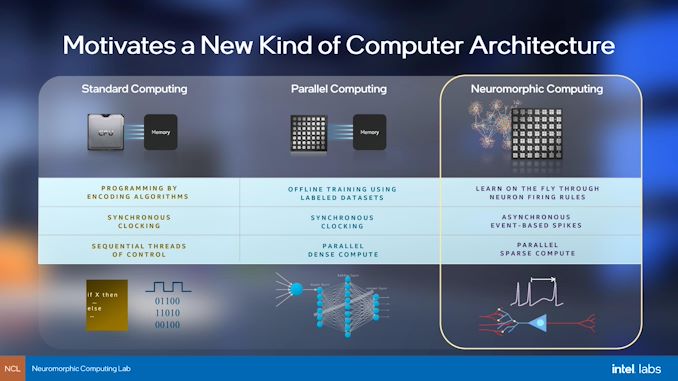
IC: Neuromorphic computing has been part of Intel Labs for almost a decade now, and it remains that way even with the introduction of Loihi 2, with external collaborations involving research institutions and universities. Is the roadmap defining the path to commercialization, or is it the direction and learnings from the collaborations that are defining the roadmap?
MD: It's an iterative process, so it's a little bit of both!
But first, I need to correct something - the acquisition I was a part of with Intel, 10 years ago, actually had nothing to do with neuromorphic computing at all. That was actually about Ethernet switches of all things! So our background was coming from the standpoint of moving data around in switches, and that's gone on to be commercialized technology inside other business groups at Intel. But we forked off and used the same kind of fundamental asynchronous design style that we had in those chips, and then we applied it to this new domain. That started about six years ago or so.
But in any case, what you're describing [on roadmaps] is really a little bit of both. We don't have a defined roadmap, given that this is about as basic of research as Intel engages in. This means that we have a kind of vision for where we want to end up – we want to bring some differentiating technologies to this domain.
So in this asynchronous design methodology, we did the best we could at Intel in developing an architecture for a chip with the best methods that we had available. But that was about as far as we could take it, as just one company operating in isolation. So that's why we released Loihi out to an ecosystem, and it's been steadily growing. We're seeing where this architecture performs really well on real workloads with collaborators, and where it doesn't perform well. There has been surprises in both of those categories! So based on what we learn, we're advancing the architecture, and that is what has led us to this next generation.
So while we're also looking for possible near term applications, which may be specializations of this general purpose design that we're developing, long term we might be able to incorporate designs into our mainstream products hidden away, in ways that maybe a user or a programmer wouldn't have to worry that they are present in the chip.
IC: Are you expecting institutions with Loihi v1 installed to move to Loihi v2, or does v2 expand the scope of potential relationships?
MD: In pretty much all respects, Loihi 2 is superior to Loihi v1. I expect that pretty quickly these groups are going to transition to Loihi 2 as soon as we have the systems and the materials available. Just like with Loihi 1, we're starting at the kind of the small scale - single chip / double chip systems. We built a 768 chip system with Loihi 1, and the Loihi 2 version of that will come around in due course.
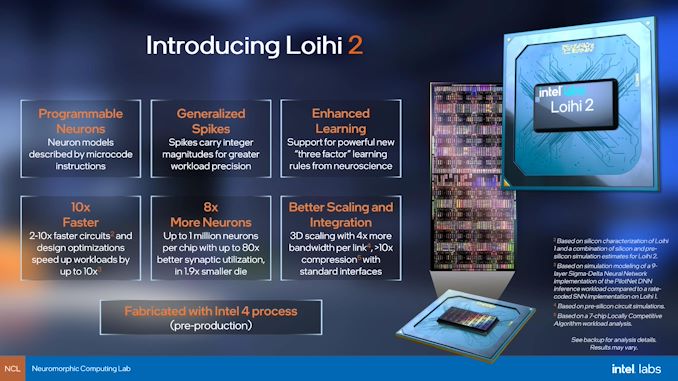
IC: Loihi 2 is the first processor publicly confirmed for Intel's first EUV process node, Intel 4. Are there any inherent advantages to the Loihi design that makes it beneficial from a process node optimization point of view?
MD: Neuromorphic Computing, more so than pretty much any other types of computer architecture, really needs Moore's law. We need tiny transistors, and we need tiny storage elements to represent all the neural and the synaptic states. This is really one of the most critical aspects of the commercial economic viability of this technology. So for that reason, we always want to be on the very bleeding edge of Moore's law to get the greatest capacity in the network, in a single chip, and not have to go to 768 chips to support a modest size workload. So that's why, fundamentally, we're at the leading edge of the process technology.
EUV simplifies the design rules, which actually is really great for us because we've been able to iteratively advance the design. We’ve been able to quickly iterate test chips and as the process has been evolving, we've been able to evolve the design and loop feedback from the silicon teams, so it's been great for that.
IC: You say pre-production of Intel 4 is used - how much is there silicon in the lab vs simulation?
MD: We have chips in the lab! In fact, as of September 30th, they'll be available for our ecosystem partners to actually kick the tires and start using them. But as always, it's the software that's really the slower part to come together. So that being said, we’re not at the final version. This process (Intel 4) is still in development, so we aren't really seeing products. Loihi 2 is a research chip, so there's a different standard of quality and reliability and all these factors that go into releasing products. But it certainly means that the process is healthy enough that we can deploy chips and put them on subsystem boards, and remotely access them, measure their performance, and make them available for people to use. My team has been using these for quite some time, and now we're just flipping the switch and saying our external users can start to use them. But we have a ways to go, and we have more versions of Loihi 2 in the lab - it's an iterative process, and it continues even with this release.
IC: So there won't specifically be one Loihi 2 design? There may be varying themes and features for different steppings?
MD: For sure. We've frozen the architecture in a sense, and we have most of the capabilities all implemented and done. But yes, we're not completely done with the final version that we can deploy with the all the final properties we want.
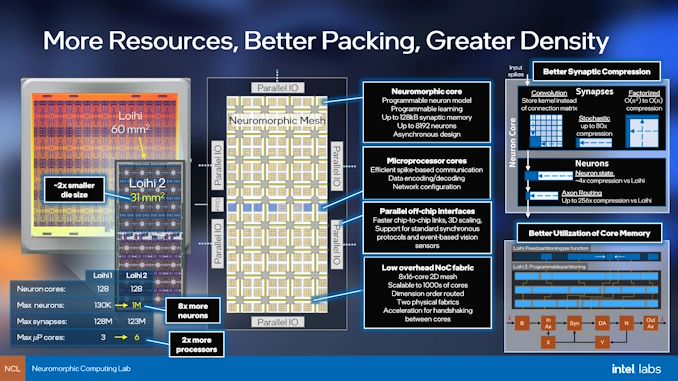
IC: I think the two big specifications that most of our readers will be interested in is the die size – going down from 60mm2 in Loihi 1 to 31 mm2 in Loihi 2. Not only that, but neuron counts increase from 130,000 to a million. What else does Loihi 2 bring to the table?
MD: So the biggest change is a huge amount of programmability that we've added to the chip. We were kind of surprised with the applications and the algorithms that started getting developed and quantified with Loihi we found that the more complex the neuron model got, the more application value we could measure. So could we could see that there was a school of thought that the particular kind of neural characteristics of the neuron model don't matter that much - what matters more is the parallel assembly of all these neurons, and then that emergent behavior I was describing earlier.
Since then, we've found that the fixed function elements in Loihi have proved to be a limitation for supporting a broader range of applications or different types of algorithms. Some of these get pretty technical but as an example, one neuron model that we wanted to support (but couldn't) with Loihi is an oscillatory neuron model. When you kick it with one of these events or spikes, it doesn't just decay away like normal, but it actually oscillates, kind of like a pendulum. This is thought in neuroscience to have some connection to the way that we have brain rhythms. But in the neuromorphic community, and even in neuroscience, it's not been too well understood exactly how you can computationally use these kind of exotic oscillating neuron models, especially when adding extra little nonlinear mathematical terms which some people study.
So we were exploring that direction, and we found that actually there are great benefits and we can practically construct neural networks with these interesting new bio-inspired neuron models. They effectively can solve the same kind of problems [we’ve been working on], but they can shrink the size of the networks and the number of parameters to solve the same problems. They're just the better model for the particular task that you want to solve. It's those kind of things where, as we saw more and more examples, we realized that it is not a matter of just tweaking the base behavior in Loihi - we really had to go and put in a more general purpose compute, almost like an instruction set and a little microcode executer, that implements individual neurons in a much more flexible way.
So that's been the big change under the hood that we've implemented. We've done that very carefully to not deviate from the basic principles of neuromorphic architectures. It's not a von Neumann processor or something - there's still this great deal of parallelism and locality in the memory, and now we have these opcodes that can get executed so we don't compromise on the energy efficiency as we go to these more complex neuron models.
IC: So is every neuron equal, and can do the same work, or is this functionality split to a small sub-set per core?
MD: All neurons are equal. In Loihi v1, we had one very configurable neuron model - each individual neuron could kind of specify different parameters to be customized to that particular part of the network, and there were some constraints on how diverse you could configure it. The same idea applies, but you can define a couple different [schema], and different neurons can reference and use those different styles in different parts of the network.
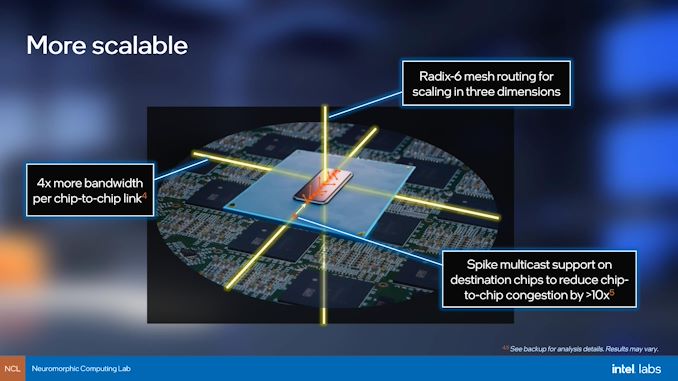
IC: One of the big things about Loihi v1 was that it was a single shiny chip which could act on its own, or in Pohoiki Springs there would be 768 chips all in a box. Can you give examples of what sort of workloads run on that single chip, versus the bigger systems? And does that change with Loihi 2?
MD: Fundamentally the kinds of workloads don't necessarily change - that's one of the interesting aspects of neuromorphic architecture. It's similar enough to the brain such that with more and more brain matter the particular types of functions and features that are supported at these different scales don't change that much. For example, one workload we demonstrated is a similarity search function – such as an image database. You might think of it as giving it an example image and you want to query to find the closest match; in the large system, we can scale up and support the largest possible database of images. But on a single chip, you perhaps performed the same thing, just with a much smaller database. And so if you're deploying that, in an edge device, or some kind of mobile drone or something, you may be very limited in a single chip form factor to the types of the varied range of different objects that it could be detected. If you're doing something that's more data center oriented, you would have a much richer space of possibility there.
But this is one area we've improved a lot – in Loihi v1, the effect of bandwidth between the chips proved to be a bottleneck. So we did get congestion, despite this highly sparse style of communication. We're usually not transmitting, and then we only transmit infrequently when there's information to be processed. But the bandwidth offered by the chip-to-chip links in Loihi was so much lower than what we have inside the chip that inevitably it started becoming a bottleneck in that 768 chip system for a lot of workloads. So we've boosted that in Loihi to over 60 times, actually, if you consider all the different factors of the raw circuit speeds, and the compression features we've added now to reduce the need for the bandwidth and to reduce redundancy in that traffic. We've also added a third dimension, so that now we can scale not just planar networks, 2D meshes of chips, but we can actually have radix, and scaling so that we can go into 3D.
IC: With Loihi 2, you're moving some connectivity to Ethernet. Does that simplify some aspects because there's already deep ecosystem based around Ethernet?
MD: The Ethernet is to address another limitation of a different kind that we see with neuromorphic technology. It's actually hard to integrate it into conventional architectures. In Loihi 1, we did a very purist asynchronous interconnect - one that allows us to scale up to these big system sizes that enables, just natively speaking, asynchronous spikes from chip-to-chip. But of course at some point you want to interface this to conventional processors, with conventional data formats, and so that's the motivation to go and put in a standard protocol in there that that allows us to stream standard data formats. We have some accelerated spike encoding processes on the chip so that as we get real world data streams we can now convert it in a more efficient fast way. So Ethernet is more for integration into conventional systems.
IC: Spiking neural networks are all about instantaneous flashes of data or instructions through the synapses. Can you give us an indication what percentage of neurons and synapses are active at any one instant with a typical workflow? How should we think about that in relation to TDP?
MD: There is a dynamic range of power. Loihi, in a real world workload on a human timescale, would typically operate around 100 milliwatts. If you're computing something that's more abstract computationally, where you don't have to slow it down to human scales, say solving optimization problems, then it’s different. One demonstration we have is that with the German railway network we took an optimization workload and mapped it onto Loihi – for that you just want an answer as fast as possible, or maybe you have a batched up collection of problems to solve. In that case, the power can peak above one watt or so in a single Loihi chip. Loihi 2 will be similar, but we've put so many performance improvements into the design, and we’re reaching upwards of 10 times faster for some workloads. So we could operate Loihi 2 at a fairly high power level, but it’s not that much when we need it for real time/human timescale kind of workloads.
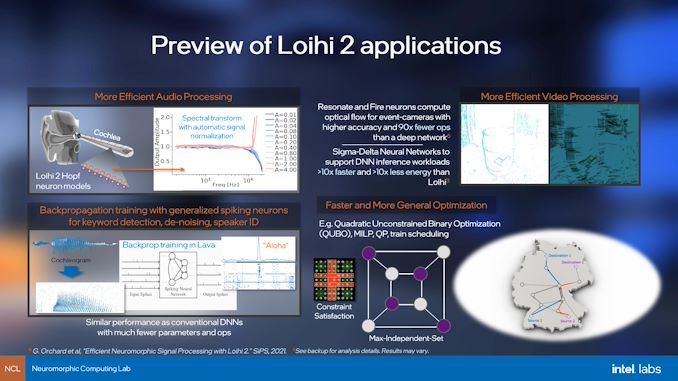
IC: In previous discussions about neuromorphic computing, one of the limitations isn't necessarily the compute from the neuromorphic processor, but finding sensors that can relay data in a spiking neural network format, such as video cameras. To what level is the Intel Neuromorphic team working on that front?
MD: So yes, there’s a definite need to, in some cases, rethink sensing all the way to the sensors themselves. We've seen that with new vision sensors, these emerging event cameras, are fantastic for directly producing spikes that go speak the language of Loihi and another neuromorphic chips. We are certainly collaborating with some of those companies developing those sensors. There's also a big space of interesting possibility there for a really tight coupling between the neuromorphic chips and the sensors themselves.
Generally though, what matters more than just the format of the spikes is that the base for the data stream has to be a temporal one, rather than static snapshots. That's the problem with a conventional camera for neuromorphic interfacing, we need more of an evolving temporal signal. So audio waveforms, for example, are great for processing.
In that case, we can look at bio-inspired approaches. For audio, this is an example where with the more generalized kind of neuron models in Loihi, we can model the cochlea (ear). In the cochlea, there is a biological structure that converts waveforms into spikes, and making a spectral transform of spikes looking at different frequencies. That's the kind of thing where that the sensor part of it, we can still use a standard microphone, but we're going to change the way that we convert these signal streams that are fundamentally time varying into these discrete spike outputs.
But yeah, sensors are a very important part of it. Tactile sensors are another example where we're collaborating with people producing these new types of tactile sensors, which clearly you want to be event based. You don't want to read out all of the tactile sensors in a single synchronous time snapshot - you want to know when you've hit something and respond immediately. So here's another example where the bio inspired approach to sensing tactile sensation is really good for a neuromorphic interface.
IC: So would it be fair to say that neuromorphic is perhaps best for interrupt based sensing, rather than polling based?
MD: In a very conventional computing mindset, absolutely! That's exactly it.
IC: How close is Loihi 2 to a 'biological model'?
MD: I think our guiding approach is to understand the principles that come from the study of neuroscience, but not to copy feature by feature. So we've added a bit of programmability into our neuron models, for example - biology doesn't have programmable neurons. But the reason we've done that is so that we can support the diversity of neuron models that we find in the brain. It's no coincidence and not a just a quirk of evolution that we have 1000s of different unique neuron types in the brain. It means that not all one size fits all. So we can try to design a chip that has 1000 different hard coded circuits, and each one is trying to mimic exactly a particular neuron - or we can say we have one general type, but with programmability. Ultimately we need diversity, that's the lesson that comes from evolution, but let's give our chip the feature set that lets us cover a range of neuron models.
IC: Is that kind of like mixing an FPGA with your model?
MD: Yeah! Actually in many ways that is the most close parallel to a neuromorphic architecture.
IC: One of the applications of Loihi has been optimization problems - sudoku, train scheduling, puzzles. Could it also be applied to combative applications, such as chess or Go? How would the neuromorphic approach differ to the 'more traditional' machine learning?
MD: That’s a really interesting direction for research that we haven't gone deeply into yet. If you look at the best performing, adversarial type of reinforcement-based learning approaches that have proven so successful there, the key is to be able to run many, many, many different trials, vastly accelerated to what a human brain could process. The algorithm then learns from all of that. This is a domain where it starts being a little distant from what we're focused on in Neuromorphic, because we're often looking at human timescales, by and large, and processing data streams that are arriving in real time and adapting to that in a way that our brain adapts.
So if we're trying to learn in a superhuman way, such as all kinds of correlations in the game of Go that human brains struggle to achieve, I could see neuromorphic models being good for that. But we're going to have to go work on that acceleration aspect, and have them speed up by vast numbers. But I think there's definitely a future direction - I think this is something that eventually we will get to, and particularly deploying evolutionary approaches for that where we can use vast parallelism similar to how in nature it evolves different networks in a kind of distributed adversarial game to evolve the best solution. We can absolutely apply those same techniques, neuromorphically, and that would be a guiding motivation to build really big neuromorphic systems in the future - not to achieve human brain sales, but to go well beyond human brain scale, to evolve into the best performing agent.
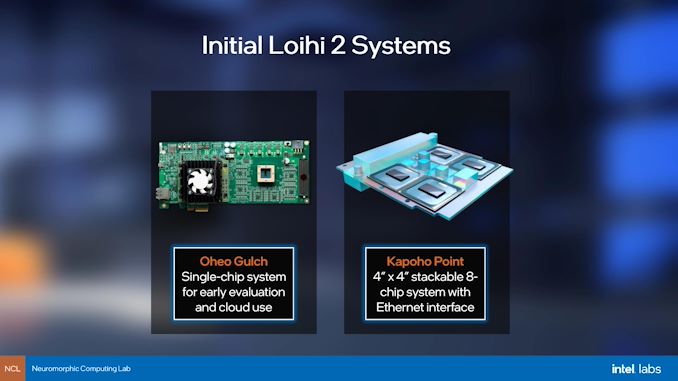
IC: In normal computing, we have the concept of IPC - instructions per clock. What's the equivalent metric in Neuromorphic computing, and how does Loihi 2 compare to Loihi 1?
MD: That’s a great question, and it gets into some nuances of this field. There are metrics that we can look at, things like the number of synaptic operations that can be processed per unit of time, or similar such as max per second, or the max per second per watt, or synaptic energy, neuron updates per time step, or per unit of time, and the numbers of neurons that could be updated. In all of those metrics, we've improved Loihi 2 to generally by at least a factor of two faster. As I was saying earlier, it's uniformly better by a big step over Loihi 1.
Now on the other hand, we tend to not really emphasize (at least in our research programme) those particular metrics, because once you start fixating on specific ops and try to optimize for them, you're basically accepting the fact we know what the field wants, and let's go optimize for those. But in the neuromorphic domain, that there's just no clarity yet on exactly what is needed. For a deep learning accelerator, you want to crank the greatest number of operations per second, right? But in the neuromorphic world, a synaptic operation, if you take something as simple as that, should that operation support the propagation delay, which has another parameter? Should it allow the weight that it applies to multiply with a strength that comes along with that spike event? Should the weight evolve in response? Should it change for learning purposes? These are all questions that we're looking at. So before we really fixate on a particular number, we want to really figure out what the right operations are.
So as I say, we've improved certainly Loihi 2 over Loihi 1 by large measures. But I think energy is an example of one that we haven't aggressively optimized. Instead, we've chosen to augment with programmability and speed, because generally what we found with Loihi is that we got huge energy gains purely from the sparsity from the activity and the architecture aspects of the design. At this point, we don't need to take a 1000x improvement and make it 2000x: for this stage of development, 1000x is good enough if we can focus on other benefits. We want balance the benefits a little bit more towards the versatility.
IC: One of the announcements today is on software - you said in our briefing earlier today that there is no sort of universal collaborative framework for neuromorphic computing, and that everybody is kind of doing their own homespun things. Today Intel is introducing a new Lava framework, because traditional TensorFlow/PyTorch or that sort of machine learning doesn't necessarily translate to the neuromorphic world. How is Intel approaching industry collaboration for that standard? Also, will it become part of Intel's oneAPI?
MD: So there are components of Lava we might incorporate into oneAPI, but really with Lava, the software framework that we're releasing, is that it's a beginning of an open source project. It's not the release of some finished product that we're sharing with our partners - we've set up a basic architecture, and we've contributed some software assets that we've developed from the Loihi generation. But really, we see this as building on the learnings of this previous generation to try to provide a collaborative path forward and address the software challenges that still exist and are unsolved. Some of these are very deep research problems. But we need to get more people working together on a common codebase, because until we get that, progress is going to be slow. Also, that's sometimes inevitable - you have to have different groups building on other people's work, extending it, enhancing it, and polishing it to the point that non specialists can come in take some or all of these best methods, that they may have no clue what magic neuroscientist ideas have been optimized, but just understandable libraries wrapped up to the point that they can be applied. So we're not at that stage yet, and it won't be an Intel product - it's going to be an open source Lava project that Intel contributes to.
IC: Speaking on the angle of getting people involved - I know Loihi 2 is an early announcement right now. But what scope is there for Loihi 2 to be on a USB stick, and get into the hands of non-traditional researchers for homebrew use cases?
MD: There's no plan at this point, but we're looking at possibilities for scaling out the availability of Loihi 2 beyond where we are with Loihi 1. But we're taking it step by step, because right now we're only unveiling the first cloud systems that people can start to access. We'll gauge the response and the interest in Lava, and how that lowers the barriers for entry to using the technology. One aspect of Lava that I didn't mention is that people can start using this on their CPU - so they can start developing models, and it will run incredibly slowly compared to what the neuromorphic chip can accelerate, but at least if we get more people using it and this nice dynamic of building and polishing the software occurs, then that will create a motivating case to go and make the hardware more widely available. I certainly hope we get to that point.
IC: If there's one main takeaway about neuromorphic computing that people should after reading and listening to this interview, what should it be?
MD: The future is bright in this field. I'm really very excited by the results we had with that first generation, and Loihi 2 addresses very specific pain points which should just allow it to scale even better. We’ve seen some really impactful application demonstrations that were not possible with that first generation. So stay tuned – there are really fun times to come.
Many thanks to Mike Davies and his team for their time.
An Interview with Intel Lab's Mike Davies: The Next Generation of Neuromorphic Research - AnandTech